DeepMind, an AI subsidiary of Google’s Alphabet, has once again successfully applied its algorithms to a challenging scientific problem. In collaboration with the Swiss Plasma Center of the Swiss Federal Institute of Technology in Lausanne (EPFL), the UK-based company has trained a deep reinforcement learning AI to control ultra-heated plasma in an experimental nuclear fusion reactor.
one in the magazine temper nature According to the published papers, the system could help physicists better understand how nuclear fusion works and may even help accelerate the development of this dreamy unlimited source of clean energy. “This is one of the most challenging applications of reinforcement learning in a real system,” comments Martin Redmiller, a researcher at DeepMind.
In nuclear fusion, the nuclei of hydrogen atoms are compressed together to form heavier atoms such as helium – a process that also occurs on the Sun. In this process, a huge amount of energy is generated in relation to an insignificant amount of the starting material, which makes it a very efficient source of energy in principle. Nuclear fusion is cleaner and safer than fossil fuels or even conventional nuclear energy. The latter uses nuclear fission, in which the nuclei are detonated, producing radioactive waste that must be disposed of.
Fourth case: plasma
However, controlling nuclear fusion in reactors is technically very difficult. Central problem: Atomic nuclei repel each other. They can only be collected together in a reactor at extremely high temperatures, often hundreds of millions of degrees – hotter than they are in the center of the Sun. At these temperatures, a substance is neither solid, nor liquid, nor gaseous. Instead, it moves into a fourth state called plasma. It’s a very hot particle soup.
The task now is to hold the plasma together in a reactor long enough to draw energy from it. Inside the Sun, plasma is held together by gravity. On Earth, physicists and chemists have to use a variety of tricks. In a magnet-based reactor, called a tokamak, plasma is trapped in a magnetic cage. Horizontal conductor rings surround the reactor vessel in the form of a circular cake, in the center of which is an elongated screw magnet that induces a ring-shaped current in the reactor and thus increases the heating of the plasma. By controlling the current in the coils, the surrounding magnetic fields can be formed. This prevents the plasma from coming into contact with the walls of the reactor vessel, which could cool it and damage the reactor at the same time.
But controlling the plasma requires constant monitoring and changing of the magnetic field, which earlier controllers managed fairly well. So the DeepMind team has meticulously trained their reinforcement learning algorithm on this in a simulation. Training with reinforcement learning should enable the software agent to act in any situation in such a way that it pursues its goal as rigorously as possible – without explicitly writing out all possible actions for all possible situations. This basically works with trial and error: it tries an action that, if effective, is more likely to be chosen next time. If they are not effective, you are less likely to be selected. After a sufficient number of attempts, the agent learns the optimal “policy”.
(picture:
UKAEA
)
Practice the virtual reactor
After the AI learned to control the shape of the plasma and change it appropriately using magnetic pulses in a virtual reactor, an experiment began. In a small experimental reactor, EPFL researchers allowed the algorithm to control the university’s Tokamak Variable Configuration (TCV). Result: It can be seen that the AI was fully able to control the real reactor without additional tuning by humans. However, the attempt was too short due to the system: the AI controlled the plasma for a total of two seconds – that’s how long the TCV could run before it heated up.
In order for the control to work, the DeepMind AI neural network records 90 different measured values about 10,000 times per second. These show, among other things, the current shape and location of the plasma. The 19 TCV magnet voltage is then configured accordingly. The resulting feedback loop runs much faster than the previously used PID controller. However, it is above all more flexible, as it allows a variety of plasma configurations to be created and controlled with only one control architecture.
To speed up the system, the AI was divided into two neural networks. In the simulation, a large network first learned how to control the reactor. These capabilities were then transferred to a smaller, faster network that controlled the reactor itself (the so-called “critic/actor” model). However, since the simulation used to train the program becomes very imprecise in certain parameter ranges, the control program excludes such “unconfirmed regions” as a precaution.
Artificial intelligence to better experience plasma controls
However, the success is impressive. “It’s an incredibly powerful technology,” says Jonathan Citrien of the Netherlands Institute for Fundamental Energy Research, who is familiar with the study. “It’s an important first step in a very exciting direction.” The research team believes that using AI to control the plasma could also make it easier to experiment with different conditions in such reactors. This in turn helps to better understand the process and possibly accelerate the development of commercial nuclear fusion reactors.
Research is progressing, but only slowly. Only recently, researchers using the JET experimental fusion reactor have shown that they can generate a self-sustaining fusion reaction with deuterium and tritium. However, in order to create a tokamak fusion reaction that produces more energy than what is put into it, a large reactor such as ITER with superconducting magnets is needed. However, it won’t be ready until 2035 at the earliest. It remains to be seen if the private companies, which are also working on smaller fusion reactors and in some cases follow completely different physics concepts, are actually faster. For now, the race is still fully open.
However, the fun of experimenting can be increased. “By using these types of control systems, we can take risks that might otherwise be a problem,” says Ambrogio Fasoli, director of the Swiss Plasma Center and president of Eurofusion. Human operators often do not want to pressurize the plasma beyond certain limits. “There are events that we must completely avoid because they damage the device.” However, if you are sure that you have a control system that does not overburden the technology despite pushing it to its limits, you can also explore completely new possibilities. “We can speed up the search.”
(JLE)
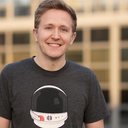
Lifelong foodaholic. Professional twitter expert. Organizer. Award-winning internet geek. Coffee advocate.